Transition path theory¶
%pylab inline
import pyemma.plots as mplt
import pyemma.msm as msm
Five-state toy model¶
The only thing we need to start with is a transition matrix. Usually, such a transition matrix has been estimated as a Markov model from simulation data (see Emma’s msm.estimation package). Here we will just define a little five-state toy model in order to illustrate how transition path theory works:
# Start with toy system
P = np.array([[0.8, 0.15, 0.05, 0.0, 0.0],
[0.1, 0.75, 0.05, 0.05, 0.05],
[0.05, 0.1, 0.8, 0.0, 0.05],
[0.0, 0.2, 0.0, 0.8, 0.0],
[0.0, 0.02, 0.02, 0.0, 0.96]])
M = msm.markov_model(P)
pos = np.array([[2.0,-1.5],[1,0],[2.0,1.5],[0.0,-1.5],[0.0,1.5]])
mplt.plot_markov_model(M, pos=pos);
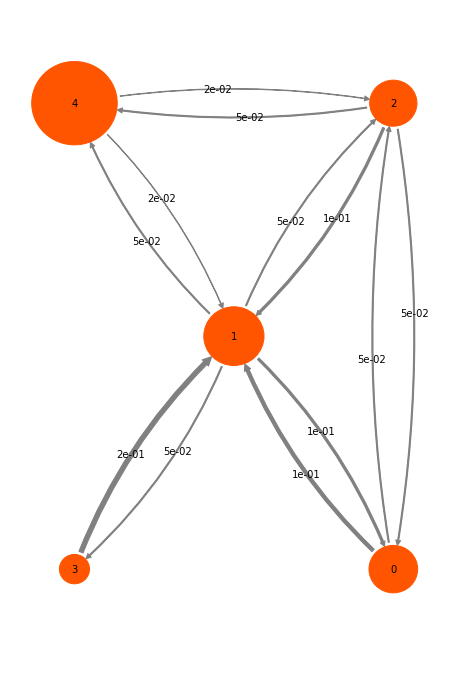
The disks represent the five discrete states, with areas proportional to the stationary probability of each state. The labels 0-4 plotted within disks are just indexes that will be used to refer to states. The arrows represent transitions between states, with their thickness proportional to the transition probability
Transition path theory¶
For doing tpt with the function msm.analysis.tpt(), we only need to pass the transition matrix and the source or reactant state A, and the product or target state B. Here we choose A=0 and B=4:
A = [0]
B = [4]
tpt = msm.tpt(M, A, B)
#tpt = msmflux.tpt(P, A, B)
The object we get back is a TPT flux. It contains a number of quantities of interest. A quantity that we are normally not so much interested in is the gross flux of the A->B reaction. The Gross A->B flux is the probability flux of trajectory that start in A and end up in B. Compared to the equilibrium flux \(\pi_i p_{ij}\), this flux is smaller because a large part (generally the vase majority) of the equilibrium flux is just spend on nonreactive trajectories that never reach B.
# get tpt gross flux
F = tpt.gross_flux
print('**Flux matrix**:')
print(F)
print('**forward committor**:')
print(tpt.committor)
print('**backward committor**:')
print(tpt.backward_committor)
# we position states along the y-axis according to the commitor
tptpos = np.array([tpt.committor, [0,0,0.5,-0.5,0]]).transpose()
print('\n**Gross flux illustration**: ')
mplt.plot_flux(tpt, pos=tptpos, arrow_label_format="%10.4f", attribute_to_plot='gross_flux')
(<Figure size 864x864 with 1 Axes>, array([[ 0. , 0. ],
[ 0.35714286, 0. ],
[ 0.42857143, 0.5 ],
[ 0.35714286, -0.5 ],
[ 1. , 0. ]]))
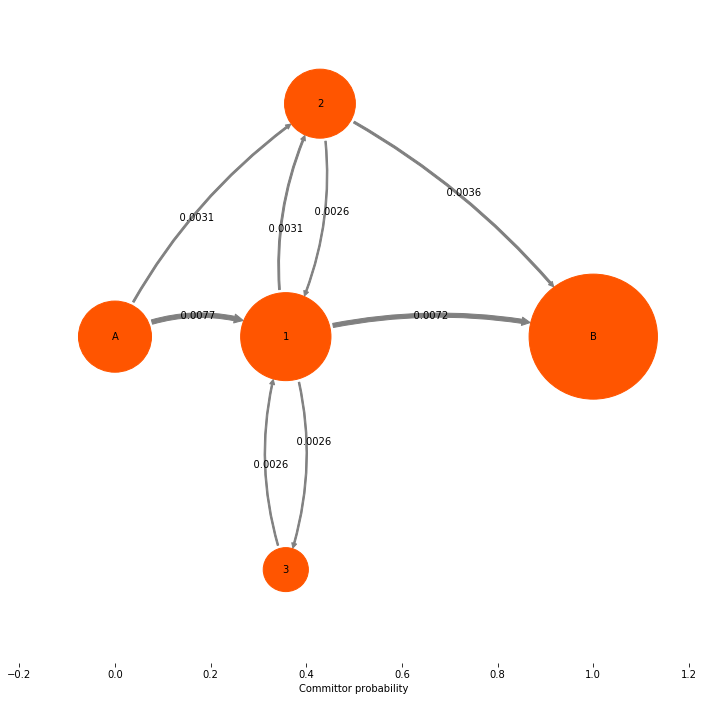
The net flux¶
Now we look at the quantity that is actually interesting: the net flux. Note that in the gross flux above we had fluxes between states 1 and 3. These fluxes just represent that some reactive trajectories leave 1, go to 3, go then back to 1 and then go on to the target state 4 directly or via state 2. This detour via state 3 is usually of no interest, and their contribution is already contained in the main paths 0->1 and 1->{2,4}. Therefore we remove all nonproductive recrossings by taking the difference flux between pairs of states that have fluxes in both directions. This gives us the net flux. The net flux is already computed by the TPT object, so normally you will just construct the TPT object and directly get the net flux from it:
# get tpt net flux
Fp = tpt.net_flux
# or: tpt.flux (it's the same!)
print('**Net-Flux matrix**:')
print(Fp)
# visualize
mplt.plot_flux(tpt, pos=tptpos, arrow_label_format="%10.4f", attribute_to_plot='net_flux')
(<Figure size 864x864 with 1 Axes>, array([[ 0. , 0. ],
[ 0.35714286, 0. ],
[ 0.42857143, 0.5 ],
[ 0.35714286, -0.5 ],
[ 1. , 0. ]]))
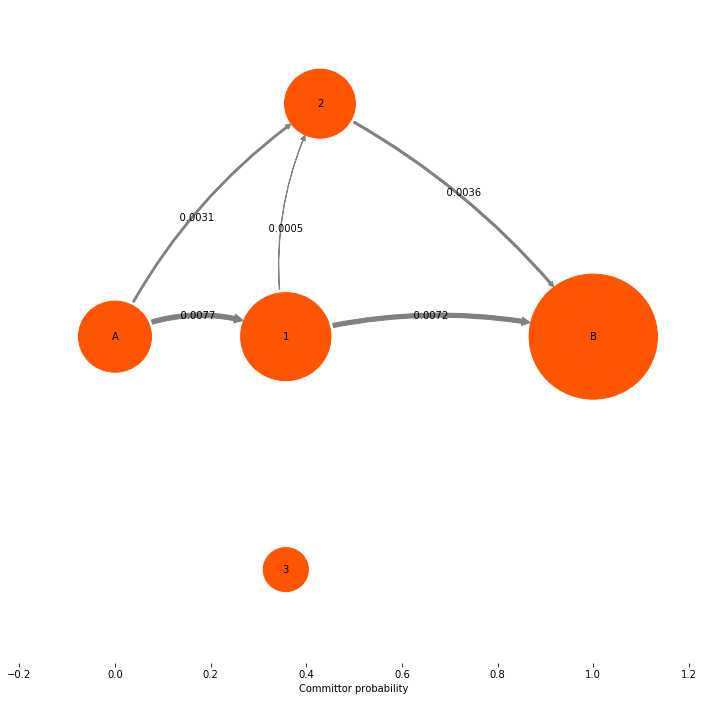
print('Total TPT flux = ', tpt.total_flux)
print('Rate from TPT flux = ', tpt.rate)
print('A->B transition time = ', 1.0/tpt.rate)
print('mfpt(0,4) = ', M.mfpt(0, 4))
In our opinion the most useful information conveyed by a TPT flux analysis are not the absolute numbers, but the mechanism, i.e. which pathways is the system going to take from A to B, and what is their relative contribution to the overall reactive flux. Therefore we may want to get rid of the absolute flux numbers and just normalize them to the total flux, so that we can talk about percentages of taking this or that pathway. Let’s do that:
mplt.plot_flux(tpt, pos=tptpos, flux_scale=100.0/tpt.total_flux, arrow_label_format="%3.1f")
ylabel("committor")
Text(0,0.5,'committor')
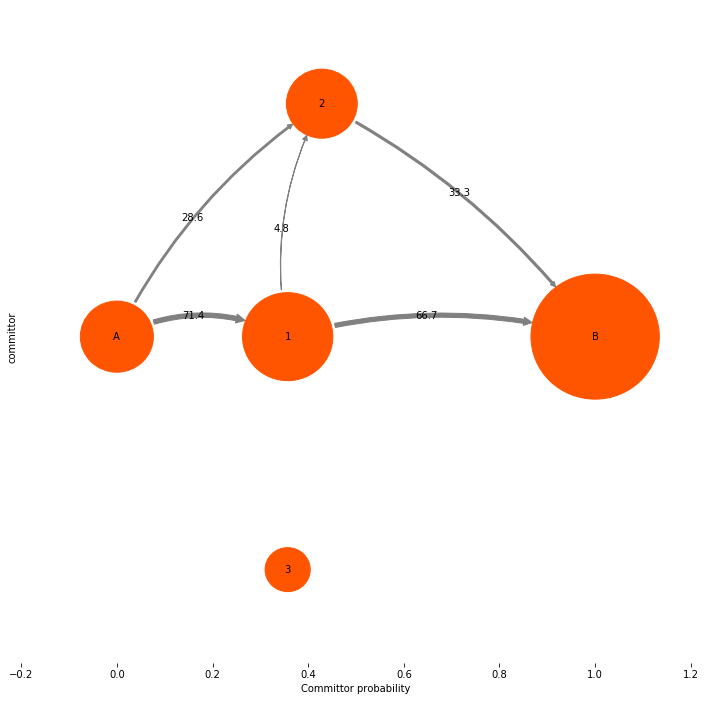
Pathway decomposition and sub flux¶
Now let us have a look how the pathway decomposition works. It’s really simple in this example. Here we just call the pathways function, and then list the pathways we have found:
tpt.pathways()
([array([0, 1, 4], dtype=int32),
array([0, 2, 4], dtype=int32),
array([0, 1, 2, 4], dtype=int32)],
[0.007203389830508427, 0.0030871670702178975, 0.0005145278450363151])
(paths,pathfluxes) = tpt.pathways()
cumflux = 0
print("Path flux\t\t%path\t%of total\tpath")
for i in range(len(paths)):
cumflux += pathfluxes[i]
print(pathfluxes[i],'\t','%3.1f'%(100.0*pathfluxes[i]/tpt.total_flux),'%\t','%3.1f'%(100.0*cumflux/tpt.total_flux),'%\t\t',paths[i])
(bestpaths,bestpathfluxes) = tpt.pathways(fraction=0.95)
cumflux = 0
print("Path flux\t\t%path\t%of total\tpath")
for i in range(len(bestpaths)):
cumflux += bestpathfluxes[i]
print(bestpathfluxes[i],'\t','%3.1f'%(100.0*bestpathfluxes[i]/tpt.total_flux),'%\t','%3.1f'%(100.0*cumflux/tpt.total_flux),'%\t\t',bestpaths[i])
Fsub = tpt.major_flux(fraction=0.95)
print(Fsub)
Fsubpercent = 100.0 * Fsub / tpt.total_flux
mplt.plot_network(Fsubpercent, pos=tptpos, state_sizes=tpt.stationary_distribution, arrow_label_format="%3.1f")
(<Figure size 864x864 with 1 Axes>, array([[ 0. , 0. ],
[ 0.35714286, 0. ],
[ 0.42857143, 0.5 ],
[ 0.35714286, -0.5 ],
[ 1. , 0. ]]))
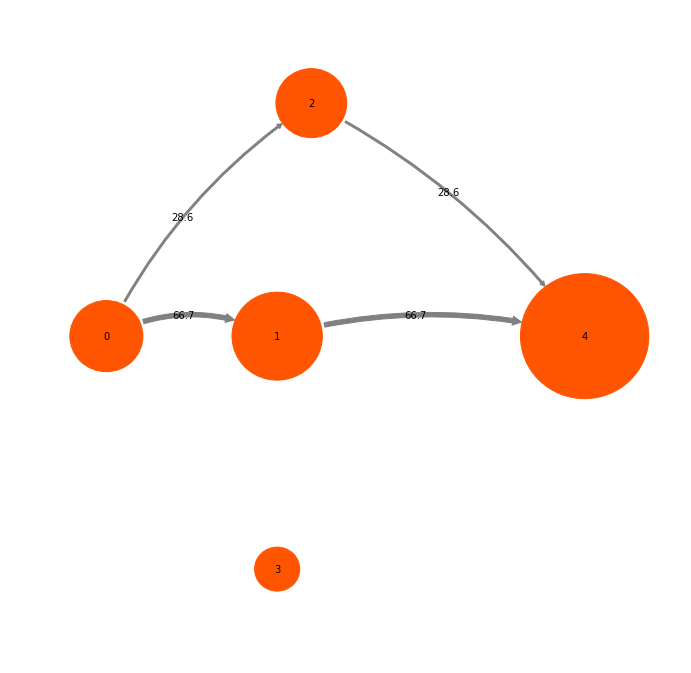
In this case the sub flux only consists of two pathways.
Coarse-graining of fluxes¶
Finally, we will look into coarse-graining of fluxes. You will need this when you have large flux networks that arise from using TPT on Markov state models with many (100s, 1000s) of microstates. In is usually not illustrative to visualize path networks with so many states. Here we will learn how to coarse-grain path networks to coarse sets of states, such as metastable sets of just user-defined sets.
We start with a somewhat larger toy model containing 16 states:
P2_nonrev = np.array([
[0.5, 0.2, 0.0, 0.0, 0.3, 0.0, 0.0, 0.0, 0.0, 0.0, 0.0, 0.0, 0.0, 0.0, 0.0, 0.0],
[0.2, 0.5, 0.1, 0.0, 0.0, 0.2, 0.0, 0.0, 0.0, 0.0, 0.0, 0.0, 0.0, 0.0, 0.0, 0.0],
[0.0, 0.1, 0.5, 0.2, 0.0, 0.0, 0.2, 0.0, 0.0, 0.0, 0.0, 0.0, 0.0, 0.0, 0.0, 0.0],
[0.0, 0.0, 0.1, 0.5, 0.0, 0.0, 0.0, 0.4, 0.0, 0.0, 0.0, 0.0, 0.0, 0.0, 0.0, 0.0],
[0.3, 0.0, 0.0, 0.0, 0.5, 0.1, 0.0, 0.0, 0.1, 0.0, 0.0, 0.0, 0.0, 0.0, 0.0, 0.0],
[0.0, 0.1, 0.0, 0.0, 0.2, 0.5, 0.1, 0.0, 0.0, 0.1, 0.0, 0.0, 0.0, 0.0, 0.0, 0.0],
[0.0, 0.0, 0.1, 0.0, 0.0, 0.1, 0.5, 0.2, 0.0, 0.0, 0.1, 0.0, 0.0, 0.0, 0.0, 0.0],
[0.0, 0.0, 0.0, 0.1, 0.0, 0.0, 0.3, 0.5, 0.0, 0.0, 0.0, 0.1, 0.0, 0.0, 0.0, 0.0],
[0.0, 0.0, 0.0, 0.0, 0.1, 0.0, 0.0, 0.0, 0.5, 0.1, 0.0, 0.0, 0.3, 0.0, 0.0, 0.0],
[0.0, 0.0, 0.0, 0.0, 0.0, 0.1, 0.0, 0.0, 0.2, 0.5, 0.1, 0.0, 0.0, 0.1, 0.0, 0.0],
[0.0, 0.0, 0.0, 0.0, 0.0, 0.0, 0.1, 0.0, 0.0, 0.1, 0.5, 0.1, 0.0, 0.0, 0.2, 0.0],
[0.0, 0.0, 0.0, 0.0, 0.0, 0.0, 0.0, 0.1, 0.0, 0.0, 0.2, 0.5, 0.0, 0.0, 0.0, 0.2],
[0.0, 0.0, 0.0, 0.0, 0.0, 0.0, 0.0, 0.0, 0.3, 0.0, 0.0, 0.0, 0.5, 0.2, 0.0, 0.0],
[0.0, 0.0, 0.0, 0.0, 0.0, 0.0, 0.0, 0.0, 0.0, 0.1, 0.0, 0.0, 0.3, 0.5, 0.1, 0.0],
[0.0, 0.0, 0.0, 0.0, 0.0, 0.0, 0.0, 0.0, 0.0, 0.0, 0.2, 0.0, 0.0, 0.1, 0.5, 0.2],
[0.0, 0.0, 0.0, 0.0, 0.0, 0.0, 0.0, 0.0, 0.0, 0.0, 0.0, 0.3, 0.0, 0.0, 0.2, 0.5],
])
M2_nonrev = msm.markov_model(P2_nonrev)
w_nonrev = M2_nonrev.stationary_distribution
# make reversible
C = np.dot(np.diag(w_nonrev), P2_nonrev)
Csym = C + C.T
P2 = Csym / np.sum(Csym,axis=1)[:,np.newaxis]
M2 = msm.markov_model(P2)
w = M2.stationary_distribution
# plot
P2pos = np.array([[1,4],[2,4],[3,4],[4,4],
[1,3],[2,3],[3,3],[4,3],
[1,2],[2,2],[3,2],[4,2],
[1,1],[2,1],[3,1],[4,1]], dtype=float)
mplt.plot_markov_model(P2, pos=P2pos, state_sizes=w, arrow_label_format="%1.2f");
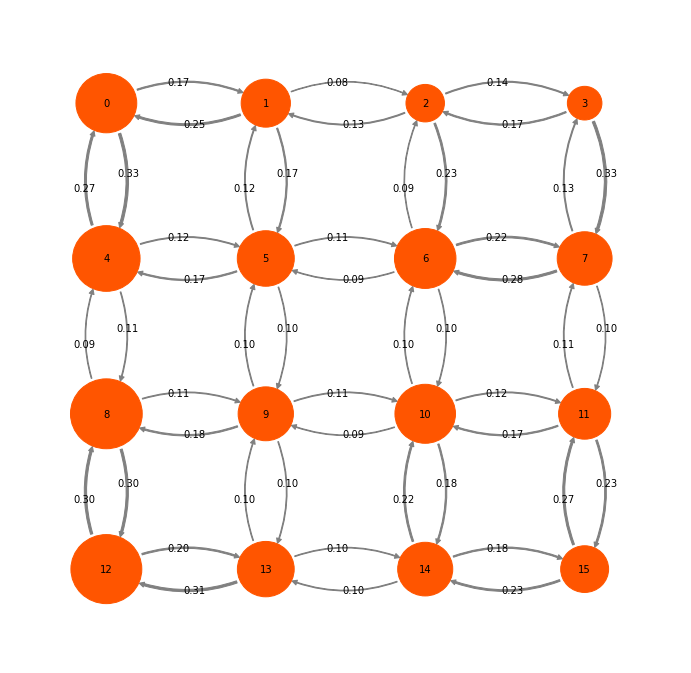
Let us first decide what a natural coarse-graining of this system would look like. Looking at the timescales, we can see that there is clear gap after three timescales (corresponding to four states). So we use PCCA to find the four most metastable sets and assign each microstate to the nearest metastable set:
def assign_by_membership(M):
return np.argmax(M, axis=1)
def clusters_by_membership(M):
a = np.argmax(M, axis=1)
m = np.shape(M)[1]
res = []
for i in range(m):
res.append(np.where(a == i)[0])
return res
print("timescales: ",M2.timescales()[0:5])
# PCCA memberships
M2.pcca(4)
M = M2.metastable_memberships
pcca_clusters = clusters_by_membership(M)
print("PCCA clusters",pcca_clusters)
The PCCA clusters are the four sets of four states in the lop left, top right, lower left and lower right corner in our illustration. So for the sake of a TPT analysis we want to lump the microstates within these PCCA clusters and only resolve the pathways connecting them.
Now we first to TPT for the full microstate transition matrix, and obtain the reactive flux in the set of 16 microstates:
# do TPT
A2 = [0,4]
B2 = [11,15]
tpt2 = msm.tpt(M2, A2, B2)
mplt.plot_flux(tpt2, attribute_to_plot='net_flux', pos=P2pos, state_sizes=w,
arrow_label_format="%1.4f", show_committor=False);
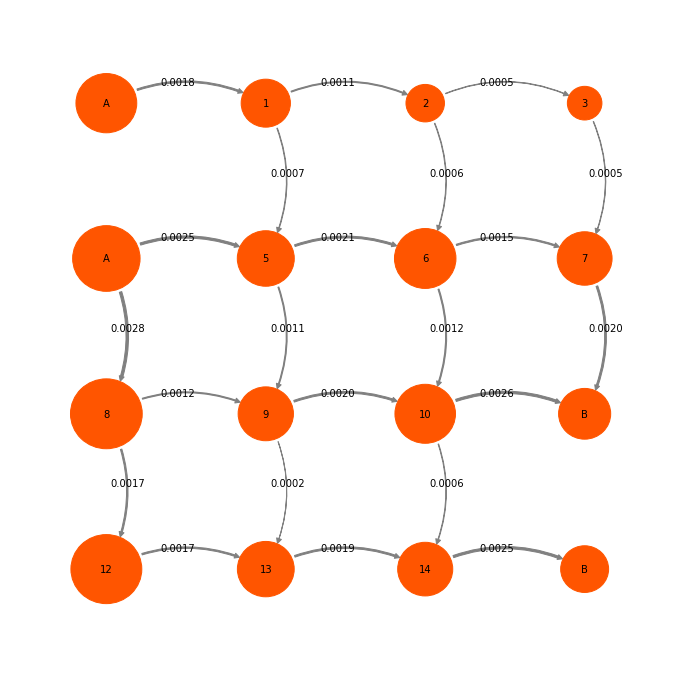
We have chosen to do TPT starting from states (0,2) and ending in states (11,15). Note that this definition is not exactly compatible with PCCA states. We have defined A and B such that PCCA states are cut. This is fine, because we usually conduct TPT in such a way as to resolve a specific process that is of our interest. Whether the sets that we want to define as TPT endstates are metastable or not is a different question. So we have to deal with this.
Now we call the coarse-grain method with the PCCA clusters we want to distinguish
(tpt_sets, tpt2_coarse) = tpt2.coarse_grain(pcca_clusters)
This function redefines the sets on which the TPT flux will be coarse-grained in order to meet two objectives:
- the states we want to distinguish will be distinguished
- the boundaries between A, B and the intermediates will be respected.
Because of this redefinition, you will get two objects as an output. First the actual set definition that was used, secondly the new coarse-grained flux. Let’s look at the sets:
print(tpt_sets)
You can see that the PCCA sets are still distinguished. In Addition the PCCA set (0,1,4,5) was split into (0,4), which is the new A set, and (1,5), which is an intermediate set. Likewise (10,11,14,15) was split into the intermediate set (10,14) and the B set (11,15).
Now we print the full information of the coarse-grained flux:
print(tpt_sets)
print(tpt2_coarse.A)
print(tpt2_coarse.B)
print("pi coarse: ")
print(tpt2_coarse.stationary_distribution)
print("sum = ",np.sum(tpt2_coarse.stationary_distribution))
print("committors : ")
print(tpt2_coarse.committor)
print(tpt2_coarse.backward_committor)
print("F coarse : ")
print(tpt2_coarse.gross_flux)
print("F net coarse : ")
print(tpt2_coarse.net_flux)
Indeed we have a normal ReactiveFlux object, just with less (6) states. In order to visualize what the coarse-graining has done to our flux, we will compute the positions of the coarse states as the mean of the fine states that were lumped:
# mean positions of new states
cgpos = []
print("set\t\tposition")
for s in tpt_sets:
p = np.mean(P2pos[list(s)], axis=0)
cgpos.append(p)
print(list(s),"\t\t",p)
cgpos = np.array(cgpos)
Let’s plot the coarse-grained flux network:
mplt.plot_flux(tpt2_coarse, cgpos, arrow_label_format="%1.4f", show_committor=False);
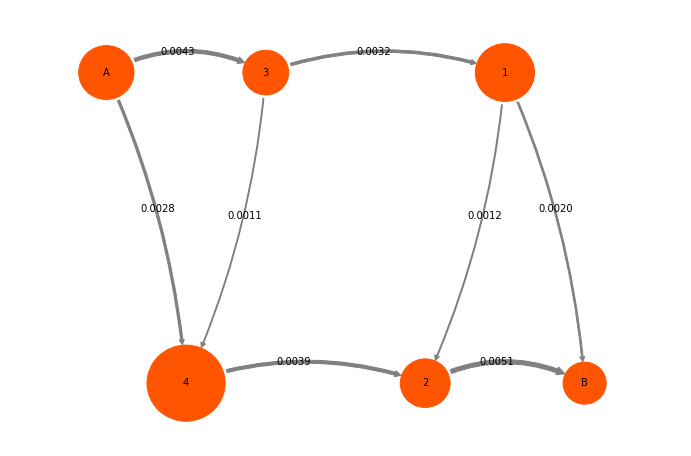
And the same as percentages:
Fpercent = 100.0 * tpt2_coarse.net_flux / tpt2_coarse.total_flux
mplt.plot_flux(tpt2_coarse, pos=cgpos, flux_scale=100.0/tpt2_coarse.total_flux, arrow_label_format="%3.1f", show_committor=False);
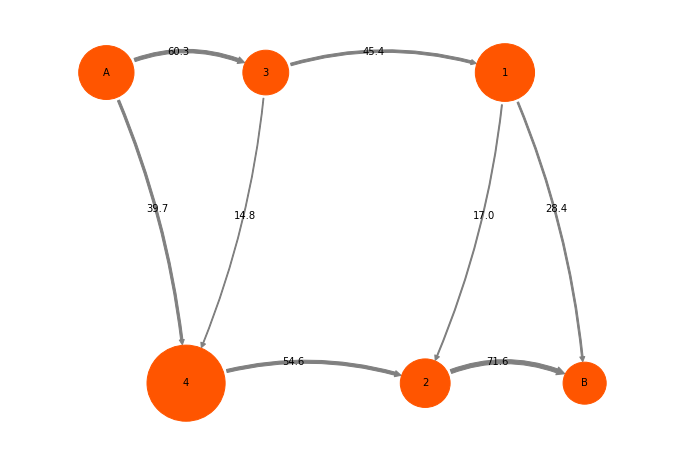
We can treat this ReactiveFlux like the microstate reactive flux. For example, we can decompose it into pathways:
(paths,pathfluxes) = tpt2_coarse.pathways()
cumflux = 0
print("Path flux\t\t%path\t%of total\tpath")
for i in range(len(paths)):
cumflux += pathfluxes[i]
print(pathfluxes[i],'\t','%3.1f'%(100.0*pathfluxes[i]/tpt2_coarse.total_flux),'%\t','%3.1f'%(100.0*cumflux/tpt2_coarse.total_flux),'%\t\t',paths[i])
Literature¶
[1] W. E and E. Vanden-Eijnden.
Towards a theory of transition paths.
J. Stat. Phys. 123: 503-523 (2006)
[2] P. Metzner, C. Schuette and E. Vanden-Eijnden.
Transition Path Theory for Markov Jump Processes.
Multiscale Model Simul 7: 1192-1219 (2009)
[3] F. Noe, Ch. Schuette, E. Vanden-Eijnden, L. Reich and T. Weikl:
Constructing the Full Ensemble of Folding Pathways from Short Off-Equilibrium Simulations.
Proc. Natl. Acad. Sci. USA, 106, 19011-19016 (2009)